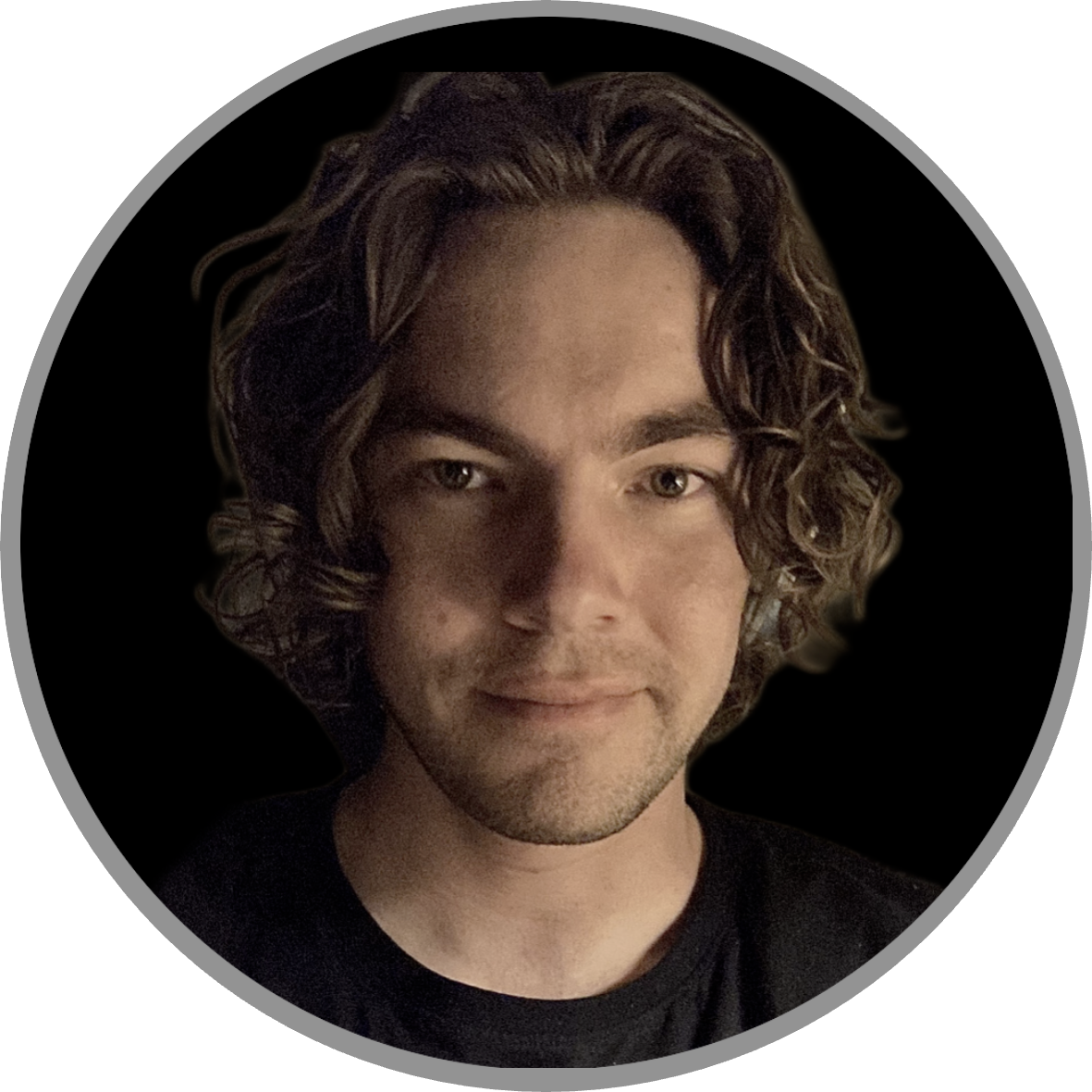
Matt Sampson
PhD candidate at Princeton University
about me
I am currently at Princeton University studying ways to advance science through the use of machine learning with a particular focus on modelling dynamical systems, representation learning, and world models. Previously I was at the Australian National University where I performed numerical experiments and helped develop a code to simulate cosmic ray propagation through a dynamically evolving turbulent plasma. I have also worked as a data scientist/computational statistician in fields of information geometry and biology.